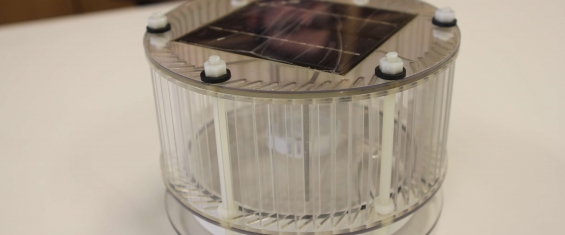
2017 IPaT Smart & Connected Communities Data Pilot Grants
The Institute for People and Technology is pleased to announce the results of our first Smart & Connected Communities Data Pilot Grant program. These pilot grants will provide funding for one semester to further interdisciplinary research within the area of Smart & Connected Communities. IPaT will support data-centric projects aiming for one or more of the following:
- Creating new forms of smart city data
- Leveraging and making available legacy city data
- Prototype targeted uses of smart city data
The result of this pilot grant program will be new collections of smart city data that can be made available to the Georgia Tech research community and new prototypes for working with that data. We received several excellent submissions, and will provide funding for six projects:
Toward Reality-Virtuality Integrated Smart Cities: Understanding Urban Scale Spatial and Temporal Dynamics of Human Interactions Through a Reality Data-Rich Virtual Atlanta
John E. Taylor, School of Civil and Environmental Engineering
Research at the city scale is limited, in part because it requires broad, societally contextualized data across spatial and temporal dimensions. Moreover, this data should necessarily incorporate the heterogeneity that exists across different cities and communities. However, as yet, we lack sufficient contextualized data to explore data relationships. We need multidimensional data analyses with characterized location embedded information. Given that individuals change their time and location designations in a heterogeneous manner, this makes explaining the root cause of such dependencies across time and space problematic at best. Locally and temporally contextualized data is needed for such an interpretation. In cities, in particular, we require deeper discovery and understanding of the way the population forms communities and interacts with the built environment. Access to urban scale high resolution contextualized spatiotemporal data is not available. An ideal opportunity for capturing contextualized data lies in the growing domain of virtual reality. Virtual Reality (VR) offers a highly effective way to simulate the spatial dimensions of urban spaces in 3D environments. By streaming reality data (e.g., individual mobility and information) into these environments, we can bring the temporal dimension into a contextualized virtual environment, extending the space-time domain to an additional context dimension. Such an environment—where real and simulated data are both spatiotemporally generated—creates exciting opportunities for real data analysis and contextualization, as well as testing simulated “what if” scenarios. Collectively, this will create opportunities for both data visualizations in immersive experiences and data exploration beyond simple numerical models.
Smart Cities Data Platform Development
Dennis Shelden, School of Architecture
The proposed initiative will develop a city data aggregation, visualization, and analytics platform for use by Georgia Tech smart city research community. The Spring 2017 effort will pilot this development by creating a web services city data aggregation platform and integrating several Atlanta city data sources into a common Web API accessible data service. A set of front-end web UI components will allow data points from the aggregated data sets to be queried and displayed – initially in a map interface. Further development will expand these initial platform access and display capabilities: expanding the supported data services to other municipal – and campus – data streams, providing 3D VR / AR interface capabilities, supporting analytics applications across multiple data streams, and providing these components and data sources to the broader Georgia Tech smart cities research community.
Sensing Traffic Conditions to Model and Predict Rider Stress
Christopher Le Dantec, School of Literature, Media, and Communication
Kari Watkins, School of Civil and Environmental Engineering
Recent work within transportation research has begun to question the accepted models for assessing cycling infrastructure. Metrics like levels of service or compatibility indexes are based on models for vehicular traffic and miss important elements of what goes into choosing one route over another. A more recent and promising model—Level of Traffic Stress—does a better job of accounting for the subjective experience of cycling; however, that model is new and would benefit from a stronger empirical foundation to clarify the transitions between the four levels of stress in the model. We propose to develop a new data set that would provide a more empirical and ground-truth foundation for modeling levels of traffic stress. To do so, we will begin prototyping and deploying purpose-built sensors to an established population of Cycle Atlanta app users in the spring of 2017 to determine which are the highest value data sources for determining the Level of Traffic Stress. To accomplish this, we will build a set of prototype IoT sensors that will complement route data collected by the cyclist tracking application Cycle Atlanta (and its derivatives used in other locations). The sensors we are looking to build will augment data collected by the app and will collect noise, air quality, and road condition data as a baseline. More importantly, we will determine which constellation of off-the-shelf sensors are needed to reliable detect object proximity and approach speed. The latter being very important to the experience of stress as near-miss and high-speed encounters with cars and trucks are the primary contributor to rider stress.
Developing a Robust Archive of Environmental Data to Support Smart Cities Initiatives
Amanda Meng, College of Computing
Ellen Zegura, College of Computing
Carl DiSalvo, Ivan Allen College of Liberal Arts
Smart cities achieve more livable and sustainable outcomes for their residents when physical and social infrastructure are connected through networked technologies. Citizens aid the city in making better service delivery and policy decisions through open and participatory data collection. Despite the recent launch of Atlanta’s smart city initiative, there is currently a dearth of data. As environmental sensors are a key component of the planned deployment of sensor technologies in Atlanta, we propose a project focused on environmental data. The goal of this project is to construct a robust archive of environmental data, from multiple sources, and make that data readily available for prototyping, and later, service development.
Making Legacy Data Available and Accessible for the Smart City
Jennifer Clark, Center for Urban Innovation
Thomas Lodato, Center for Urban Innovation
As a limited inquiry into these challenges and opportunities for legacy data, we propose exploring the process of digitizing and making accessible the budget and fiscal reporting data currently available from the City of Atlanta. The goals of this research are both practical and exploratory. Through this demonstration case, we will ascertain the cost and timeline for digitizing public data more generally as well as understand the challenges and opportunities incurred by making publicly available data operational for smart city systems through online databases and APIs. In short, the project addresses the problem of developing a process and method for converting data series, making data series available, and elaborating on policy issues related to smart cities' data stewardship.
Wind Speed and Acoustic Activity Extensions to the Tech Climate Network
Matthew Swarts, Center for Geographic Information Systems, IMAGINE Lab, IPDL
Brian Stone, Urban Climate Lab, SCaRP
Noah Posner, Center for Geographic Information Systems, IMAGINE Lab, IPDL
We propose to design, build, and deploy a complementary environmental sensor station alongside the current 28 nodes in the Tech Climate Network. The station will include an anemometer to capture wind speed data, but will also contain a low-cost, yet reliable, temperature and relative humidity sensor. To leverage the distribution of the current network across the campus, we will also integrate an acoustic sensor that can be used to detect acoustic activity within the range of the station. Not only does this allow us to extend the existing data to most thermal comfort models, but also has the potential to correlate with human and non-human activities. The station will also be solar-powered, and where available, report data in real-time through the existing GT Wi-Fi network. The current nodes are battery powered and data must be manually collected every 1-2 months.