IC Researchers Earn 2018 IJRR Paper of the Year for Impactful Robotics Research
Jun 28, 2019 — Atlanta, GA
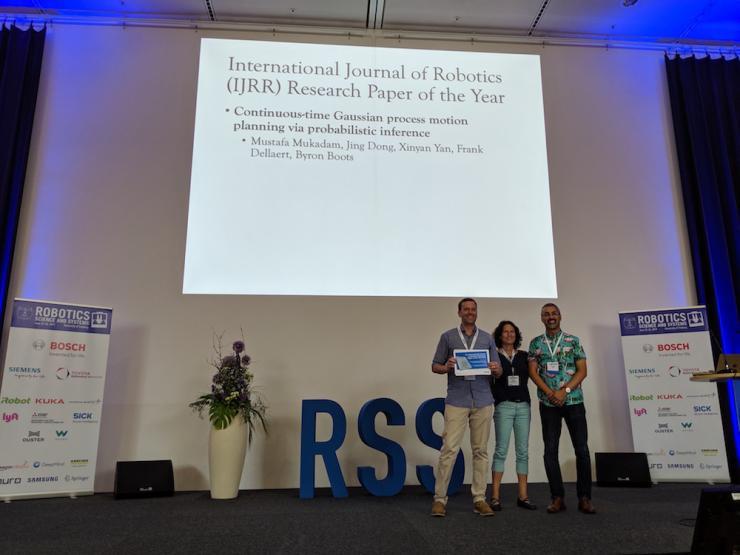
A paper published in the International Journal of Robotics Research (IJRR) by researchers in the School of Interactive Computing (IC) was selected as the 2018 IJRR Paper of the Year.
Chosen from a shortlist considered by the IJRR Executive Committee, the paper, Continuous-time Gaussian Process Motion Planning via Probabilistic Inference, was recognized for its technical rigor, relevance, and potential for impact in the robotics research community. The research comes from IC Ph.D. students Mustafa Mukadam and Jing Dong, master’s student Xinyan Yan, and advisors Professor Frank Dellaert and Assistant Professor Byron Boots.
This paper introduces a novel formulation of motion planning that treats the problem of finding an efficient, feasible path between two points as probabilistic inference with Gaussian Processes. Motion planning is a hard problem, and state-of-the art sampling-based and trajectory optimization algorithms have well-known drawbacks. The former can effectively find feasible trajectories but often exhibits jerky and redundant motion, and the latter requires a fine approximation of the trajectory to reason about thin obstacles or tight constraints.
In their paper, the team of researchers adopts a continuous-time representation of trajectories, viewing them as functions that map time to robot state. Combing this representation with fast approaches to probabilistic inference, they developed a computationally-efficient gradient-based optimization algorithm called a Gaussian Process Motion Planner that can overcome large computational costs associated with fine discretization, while still maintaining smoothness of motion in the result.
With the award comes a $1,000 prize. Boots attended the Robotics: Science and Systems (RSS) conference in the Freiburg, Germany, this week, where he accepted the award on behalf of his team.
Another paper involving Boots was also awarded a Best Student Paper Award at RSS. Titled An Online Learning Approach to Model Predictive Control, the paper was written by Robotics Ph.D. students Nolan Wagener, Ching-An Cheng, and Jacob Sacks, along with Boots.
It shows that there exists a close connection between model predictive control (MPC), a popular technique for solving dynamic control tasks, and online learning, an abstract theoretical framework for analyzing online decision making. This new perspective provides a foundation for leveraging powerful online learning algorithms to design MPC algorithms. Toward this end, the researchers propose a generic framework for synthesizing new MPC algorithms called Dynamic Mirror Decent Model Predictive Control.
The framework exposes key design choices that can help practitioners easily develop new control algorithms tailored to the challenges of their specific task. The approach is validated by developing new MPC algorithms that consistently match or outperform the state-of-the-art on several tasks including an aggressive driving problem with the goal of racing an autonomous car around a dirt track under computational resource constraints.
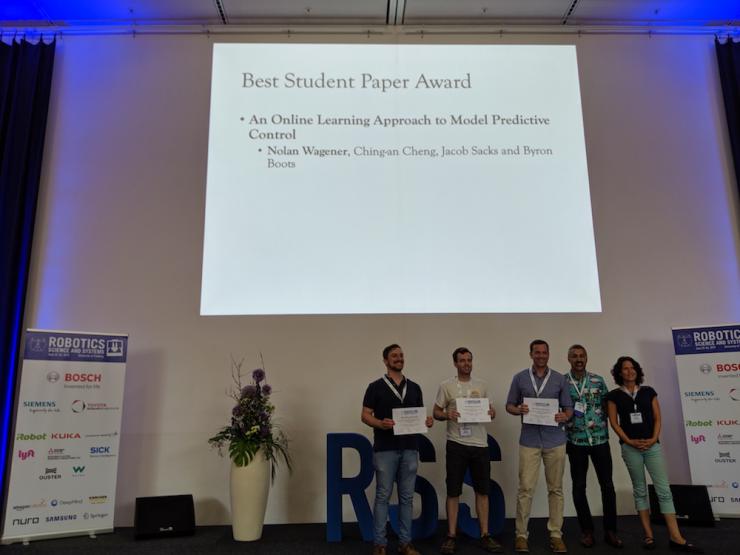